Researchers are finding why neural networks work best in their predictions
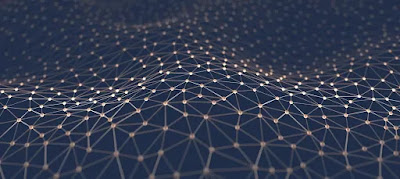
Poor intelligence, machine learning and neural networks words are widely used in everyday life.
Face recognition, object detection, and human classification and division of tasks are common functions of machine learning algorithms that are now widely used. At the heart of all of these processes is mechanical learning, which means that computers can capture important structures or key aspects of processes where the interaction between objects is very difficult.
The learning process includes good and bad examples that have no prior knowledge of objects or the basic laws of physics.
However, as it is a blinding process, machine learning is like a black box: computers make decisions that they consider to be valid but it is not clear why one decision is taken and not another, so the internal mechanism is not yet clear. Because of this, predictions made by machine learning in critical situations are dangerous and unreliable because the results can be misleading.
See:PUBG is among the more than 118 apps banned by Indian govt: Here is the full list
In this study, a research team composed of Vladimir Baulin, from the Department of Chemical Engineering at URV, Marc Werner (Leibniz Institute of Polymer Research in Dresden) and YachongGuo (University of Nanjing, China) tested neural network predictions to check their compatibility. So far, they have chosen a well-defined practical model: the neural network had to form a polymer molecule that would cross the lipid membrane in as short a time as possible. The lipid membrane is a natural barrier that protects cells from damage and external damage. To monitor neural network predictions, researchers have developed a novel numerical method that uses a complete calculation system that determines all probability of polymer formation by directly arranging photo cards that work well with the same numbers.
See:What Is the Difference Between Deep Learning and Learning Machines?
"A traditional computer processor can contain a maximum of 12-24 nuclei of calculation, but picture cards are designed to perform the same pixel calculations for photos and videos, and have thousands of counters designed for the same calculation," explains Vladimir Baulin. This huge calculation makes polymer compounds in thousands in seconds or just a few minutes. In this way a map can be made that contains all the possible combinations and, therefore, how the neural network selects the appropriate results can be considered.
"Surprisingly, a simple network like that of a network can detect the formation of a molecule," Baulin said. "This may be because environmental systems are compliant with the laws of nature, which are consistent and consistent. This greatly reduces the number of parameter combinations that can be captured by a neural network."
Therefore, comparing the effect of a neural network with the actual effect not only makes predictions possible but also shows how predictions change when the function is changed. And again, this shows how neural networks make decisions and how they think.
See:7 data science certificates to improve your salary continuity
neural networks,artificial neural network,deep dream,alexnet,cs231n,neural networks and deep learning,multilayer perceptron,deep neural network,
Also See:
- WhatsApp clients need to look out for perilous 'crash code' messages
- Participation may be risk in ML
- Presenting the concept of smallest AI / ML supercomputer
- Is free antivirus enough for my computer?
- 10 incredible things you can do with php in 2020
- The different about hiring data scientists in 2020
- Billion Android phones could be vulnerable to 400 Achilles
0 Comments
If you have any doubt, Please let me know.