Involvement in participation can be the next risk in machine learning
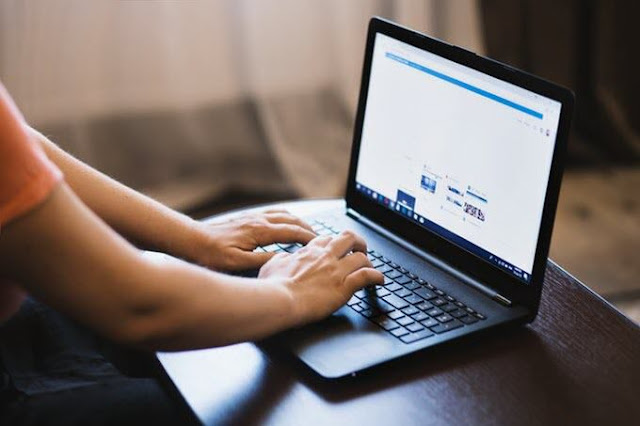
Many people simply participate in field work without being recognized or paid.
The AI community is finally awakening to the fact that machine learning can cause unimaginable harm to groups that are already oppressed and marginalized. We have activists and editors to appreciate that. Now, machine researchers and experts are looking for ways to make AI more, more accountable, and more transparent - but also, more recently, more involved.
See :
One of the most exciting and successful events at the International Electronic Conference in July was called the “Method of Collaborative Learning.” The issue has been embedded in the community's desire to build democratic, co-operative, and equitable systems through participatory processes. Such methods bring those who are in contact with and affected by the algorithmic system into the construction process - for example, asking nurses and doctors to help develop a tool for diagnosing sepsis.
This is a much-needed intervention in the field of machine learning, which may be more participatory and oral. But it’s not a silver coin: in fact, “participatory bath” can be a dangerous photo for the next field. That is what I, along with my colleagues Emanuel Moss, Olaitan Awomolo, and Laura Forlano, argue in our recent paper "Participation is not something that is designed for machine learning."
Ignoring planned stress patterns and right leads to uncontrolled and overcrowded machine learning systems. These patterns have entered the industry 30 years ago. Meanwhile, the world is watching the unprecedented growth of resource inequality and climate change driven by biodiversity. These problems are rooted in a major shift in capitalism: the background. Participation, too, is often based on the same concept of extraction, especially when it comes to machine learning.
See:
Participation is not free
Let's start with this perspective: participation is already a big part of learning about the machine, but in more complex ways. Another way is to participate as a profession.
Whether their work is acceptable or not, many participants play a key role in producing data that is used to train and test machine learning models. Photos taken and sent are spread across the web, and low-level staff at bases such as Amazon Mechanical Turk make those images personalized data. Users of the standard website also make this annotation, when filling out the reCAPTCHA. And there are many examples of so-called ghost work - the name of the anthropologist Mary Gray of all the things behind the scenes that make the programs seem to work. Many of these interactions are not well compensated, and in many cases undetected.
Participation as a consultation, meanwhile, is a practice that is evident in areas such as urbanization, and is increasingly being studied mechanically. But the effectiveness of this method is limited. The shorter the stay the longer, without the plan to establish an existing long-term relationship. Concerns about intellectual property make it difficult to truly examine these tools. As a result, this participatory approach is more likely to work.
See:
Also promising is the concept of participation as justice. Here, all the members of the creative process work together in a tightly connected relationship and constant communication. Participation as justice is a long-term commitment focused on creating products directed by people from a variety of backgrounds and communities, including the disabled community, which has long been involved here. This concept is very socially and politically important, but the capitalist market structures make it difficult to do well.
Machine learning first reaches the broader aspects of the technology industry, focusing on scale and extraction. That means the participation of the participant machine now, is oxymoron. By default, most machine learning programs have the ability to view, compress, and force (including function). These programs also have access to permissions — for example, by requiring users to log in to viewing programs in order to use certain technologies, or by using default settings that prevent them from exercising their privacy rights.
Given that, it is not surprising that machine learning fails to respond to existing forces and takes a collaborative approach. If we ignore it, learning about a participatory machine can follow the path of AI standards and become another type of face used to create injustice.
A better way
How can we avoid these dangers? There is no easy answer. But here are four suggestions:
Be careful to participate as an activity.
Most people use machine learning programs as they go about their day. Most of these activities maintain and improve these systems and are therefore important for program owners. To acknowledge this, all users must be asked for permission and given ways to opt out of any program. If they choose to participate, they should be compensated. Doing so may mean determining how and when user-generated data will be used for self-training purposes (for example, an ad on Google Maps or a sign-in notification). This would also provide appropriate presidential support for content, compensation for illegal employees, and compensation or non-monetary compensation schemes to compensate users for their personal and employee information.
Make the participation context straightforward.
Instead of trying to use one-size-fits-all approach, professionals should be aware of the specific conditions in which they work. For example, when designing a youth and group violence predictor program, experts should continually analyze the ways in which they build their own knowledge of the knowledge and technology of the environment, and work with the people who create it. This is very important as the theme of the project changes over time. Writing down or short breaks in process and context can form the basis of knowledge for long-term, effective participation. For example, should only physicians be shown in the design of a clinical care program, or should nurses and patients be included? Making it clear why and how certain communities participate makes such decisions and relationships transparent, accountable, and effective.
See:
Plan to participate for a long time from the beginning.
People are more likely to remain involved in the process later if they are able to share and receive information, as opposed to being excluded from them. This can be difficult to achieve in machine learning, especially in related design cases. Here, we have to accept the disagreements that make long-term machine participation learn about the machine, and realize that cooperation and justice are not inevitable. These values need to be adjusted regularly and should be repeated over and over again in new situations.
Learn from past mistakes.
Many injuries can be caused by repetitive thinking patterns that produced harmful technology in the first place. We as investigators need to improve our thinking ability in terms of applications and expertise. To simplify that, the mechanical and structural learning community can expand the database to highlight project failure failures (such as Sidewalk Labs' water project in Toronto). These failures can be attributed differently to socially constructive ideas (such as issues related to racial inequality). This data should teach design projects in all sectors and domains, not just those that study the machine, and clearly acknowledge their presence and externalities. These limitless cases are often the ones we can learn the most from.
It is heartwarming to see the technological community embrace questions of justice and equality. But the answers should not work by participating alone. The desire for the silver bullet has plagued the tech community for a very long time. It is time to accept the difficulties that come with the challenges of the goal of learning capitalism.
Also See:
- Top Code Sharing Websites
- As humans who have read a major area of our brain we can ‘recycle’
- Microsoft only prevented Windows 10 users from removing its Google Chrome rival
- Microsoft Edge is making it harder to dig into browsers
- Cyber resilience in the new general
- Great learning puts 25 people in leadership roles
- Facebook Gaming App on iOS is Banned by Apple's Guidelines
- Microsoft may finally have some encouraging news for Windows 10 users
- Creative Machine-Learning World GANs
- Samsung can pull the plug on Galaxy S10/Note 10 to stay profitable
- Samsung Galaxy Z Fold 2, Tab S7 and S7+, Galaxy Watch3 photos day early
- One Plus Nord on sale
0 Comments
If you have any doubt, Please let me know.