Artificial intelligence Training Method Exceeds GPT-3 Performance with 99.9% Fewer Parameters
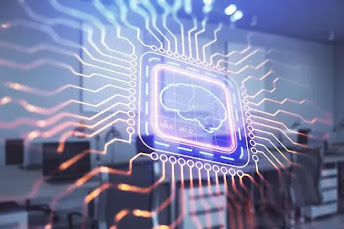
A group of researchers at LMU Munich have created Pattern-Exploiting Training (PET), a profound getting the hang of preparing method for normal language handling (NLP) models. Utilizing PET, the group prepared a Transformer NLP model with 223M boundaries that out-played out the 175B-boundary GPT-3 by more than 3 rate focuses on the SuperGLUE benchmark.
See:WhatsApp clients need to look out for perilous 'crash code' messages
PhD understudy Timo Schick and educator Hinrich Schütze of the college's Center for Information and Language Processing depicted their cycle and trial brings about a paper distributed on arXiv. PET is a procedure for adjusting a pre-prepared language model that produces extra "delicate named" preparing information from unlabeled models. This enables the model to improve execution in "few-shot" situations, for example, NLP benchmarks which have not many marked models for adjusting. Utilizing PET, the scientists calibrated an ALBERT Transformer model and accomplished a normal score of 76.8 on the SuperGLUE benchmark, contrasted with GPT-3's 71.8.
Managed AI regularly requires enormous datasets to perform well on undertakings, for example, PC vision or NLP. Nonetheless, naming these huge datasets can be tedious and costly, as it requires human laborers to physically recognize objects in pictures or rate a sentence's notion. For NLP assignments, numerous specialists have gone to move realizing, where an enormous model is pre-prepared by means of self-managed learning on a huge unlabeled dataset, for example, the substance of Wikipedia. When a model is pre-prepared, it very well may be "tweaked" for a particular assignment, for example, slant investigation, utilizing managed learning on an a lot littler marked dataset. Most best in class NLP results are accomplished by calibrating a pre-prepared Transformer model.
See:Researchers are finding why neural networks work best in their predictions
Few-shot learning is a situation identified with tweaking that tests a model's capacity to sum up to new errands, given just a couple of instances of that task - frequently less than 100, once in a while as not many as ("one-shot") or even none ("zero-shot"). OpenAI's 175B-boundary GPT-3 demonstrated that an enormous pre-prepared model could perform well in not many shot learning situations, without even calibrating the model's boundaries; rather, refreshing the model's inside state or "setting" with a literary depiction of the undertaking alongside text models was adequate to deliver "close to cutting edge results" with just 32 models. Notwithstanding, Schick and Schütze call attention to certain downsides of this methodology: limits on the setting size confine the quantity of models that can be utilized, and all the more significantly, it depends on a model that is so huge it is "usable in some true situations."
So as to accomplish comparable execution with a littler model, the analysts have created PET, a semi-administered preparing strategy that produces extra preparing information from the couple of shot models. PET works by first changing over the info models into cloze-style phrases. These are utilized to calibrate a group of language models which are then used to comment on a huge unlabeled dataset to deliver a "delicate named" dataset. The last model is then adjusted on the delicate named information. Applying PET to the SuperGLUE datasets, the group made a delicate named dataset called FewGLUE, which they used to adjust an ALBERT model that surpassed GPT-3's couple of shot execution on the SuperGLUE benchmark.
Related Articles:
- PUBG is among the more than 118 apps banned by Indian govt: Here is the full list
- What Is the Difference Between Deep Learning and Learning Machines?
- India bans PUBG, Baidu and more than 100 apps connected to China
- 7 data science certificates to improve your salary continuity
- Participation may be risk in ML
- Presenting the concept of smallest AI / ML supercomputer
- Is free antivirus enough for my computer?
- 10 incredible things you can do with php in 2020
- The different about hiring data scientists in 2020
- Billion Android phones could be vulnerable to 400 Achilles
0 Comments
If you have any doubt, Please let me know.